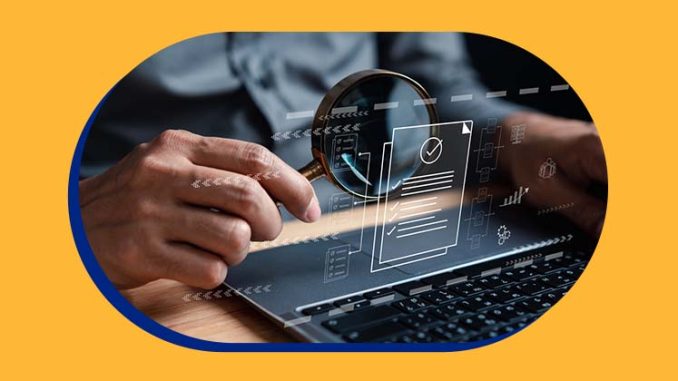
Predictive analytics has revolutionized many industries. But for the insurance industry and risk management, in this paper we present a particularly key transformative moment as it will bring improvement to an underwriting process unsuited to modern market conditions. In essence today, carriers and risk managers can make better decisions with more precision than at any previous time in history. That is, they have access to properly informed data which can be acted on quickly and accurately. This passage not only improves traditional business operations but offers a fresh perspective on risk.
Dragon Slayers with Data through Predictive Analytics Reducing Risk
There once was a large-scale enterprise into hotel people and film which had no successor or rearing method for the children born when one died In addition Disgruntled customers who posted building routines on the internet got backup for their claims while friends would join in with complaints online The arrival of predictive analytics now makes insurance decisions one of facts: how can this be? It can manage huge amounts of structured and unstructured data including historical claims records, social media activity, old census data and so on as mentioned in previous sections.
Even examine trends not currently captured in predictors (such as GDP figures for last year). Thitherto underwriters were confined to static sources of information such as one’s credit score and past claims history but now predictive models can incorporate real-time data, providing a far superior and more nuanced risk profile. The result is that pricing logic is superior with underwriting offering an improved outcome.
Now, however, predictive analytics has moved intoa forward-looking mode. By analyzing patterns and trends among customers behavior or market conditions further ahead, insurers can much better gauge what future risks might follow from today’s risks taken. Predictive models might spot early signs of likely policyholder behavior that would later result in claims by examining merchandise patterns, such as changes in work status or lifestyle events changes (major drivers and many other elements).
This leads to greater accuracy of underwriting decisions and less risk exposure from highly undesirable individuals or businesses Personalized Policies The advantages of predictive analytics in underwriting personalized policies are many. Insurance can be narrowed down to suit individual risk profiles- and gone are the days when one-size-fits-all was really the order. Drivers can now easily obtain their own pa policies from someone who works shop hours that are tailored to working people if they use telematics data and driver feedback technology in cars.
This level of personalisation is especially beneficial in fields such as auto insurance Telematics data·linked to travel habits. trip speed and frequency of travel?can be analysed in order to create custom policies for drivers in today’s cities Medical history and lifestyle habits are evaluated by the Predictive analytics employed by health insurers and life carriers in a manner that helps them to implement truly effective wellness programs.
Real-time Insurance Underwriting
In the old days, underwriting was a lengthy process. Say one may re-review policies again and even again – and over a year that might involve reviewing a few hundred times. Predictive analytics by contrast reintroduces underwriting in real time. Insurers today use machine learning techniques to process data instantly and make counter-offers on policies within minutes. This greatly speeds up underwriting processing times while customers receive faster turn-around times from the insurance company staffs as well.
Fraud Recognition and Prevention
One of the toughest problems insurers face is distinguishing legitimately from fraudulent-claime da.? Through predictive analytics, Whether a claim is genuine or not can also be determined by analyzing patterns and anomalies in claim data. Machine learning methods autonomously seek out anomalies and alert potential fraudulent activities before they cause financial losses for the insurer. These models are constantly learning from new data; over time they become more accurate, so strengthening fraud-prevention measures. By identifying abnormal behavior at the start, insurers can avoid paying out claims that are not true. This saves billions of dollars each year.
Risk Management
In the long run, there are plenty of things managers do each day: Demographics Insight (Predictive) Because of predictive analytics, risk management can be pushed forward by companies to avoid the bad from ever happening. Instead of being too big or late to change something after it’s done, predictive models are able to forecast the future market enviornment, supply chain weaknesses and geopolitical factors that may affect company processes. If these risks are taken into account in advance then companies can defend their assets, employees and other stakeholders. Everything from adjusting insurance coverage and making preemptive moves like enhancing cybersecurity or diversifying supply chains.
=Better Customer Service
Forecasting Predictive models may be able to help companies keep customers they would otherwise lose. Insurance companies use it to examine customer actions and figure out when their customers might leave. For instance, it is possible for an insurance company to determine which customers could dump them for another insurer: addsuz may occupy a range of tailored preservation programs in the form discounts, better service and also facility expansion. This is an insurance company’s competitive edge in markets that are teeming with product offerings, and it foments long-term customer relationships.
Risk Governance and Compliance Compliance is an essential element of risk management in the insurance industry. Predictive analytics can assist in ensuring business stays compliant with ongoing edicts. Businesses could eliminate human errors and avoid punitive measures by bringing compliance requirements under surveillance. For examples, predictions from models can be employed to determine the likely effect of new regulations on a company’s portfolio, so businesses don’t have to wait until tomorrow. Instead they adjust their underwriting strategies on the spot.
Predictive Analytics in Underwriting’s Future As predictive analytics matures, so it’s contribution in underwriting and risk management is growing more sophisticated all the time. With advances in AI (artificial intelligence) and IOT (internet of things), insurers have access to far finer-grained data than ever before–for health insurance is wearable technology maybe physiabox data monitoring; for property insurance corporate goods could be monitored in real time. Multipoint sensing, general insurance and object-related feelings will further enhance accuracy when it comes to assessing risk and setting premium levels.”
When block chain technologies are utilized (certainly,) another layer of dimension in essence is added to Big Data so that more areas must soon join it.On the other Synapse eliminates middlemen like insurers and reinsurers from data that is shared between them on a more transparent basis and this greatly diminishes underwriting fraud.
In conclusion tonight, we can see that the impact on underwriting and risk management by predictive analytics is significant than that of anything seen before. As it throws further light on the risk factors and permits real-time decision-making along with personalized solutions for customers, insurers are much better equipped to navigate the myriad extensiones to today’s perilous environment. When it comes to predicting insurance costs, predictive analytics is already becoming the standard tool among underwriters. Benefits–from smart underwriting that is less risky and more valuable for both insurers and policyholders–will surely accrue in time.